Is She Smiling?
Testing bias in facial expression recognition
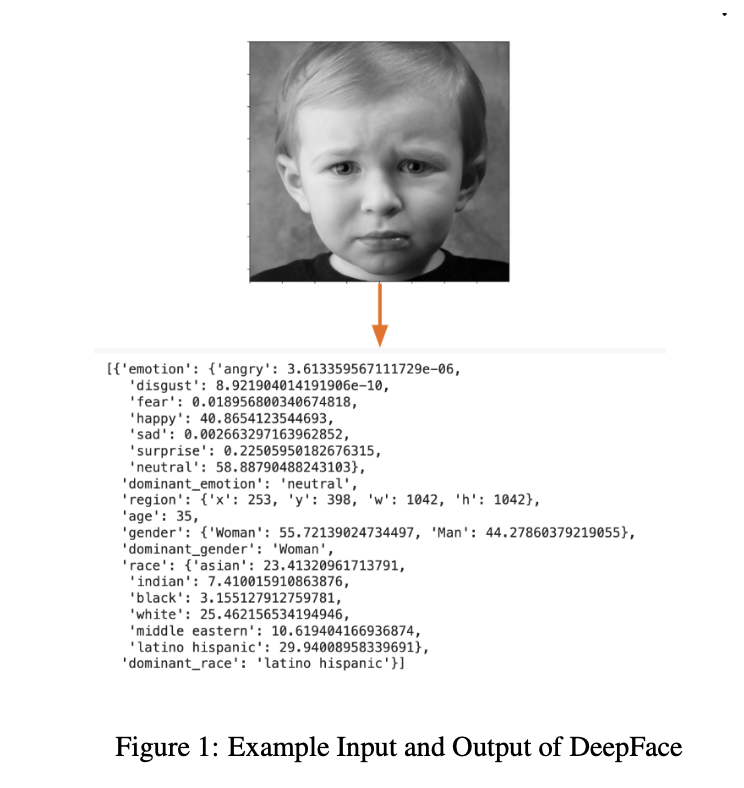
This is a project I collaborated with three other women in testing bias in AI systems. In this study, we examined the inherent biases in facial expression recognition technology. We tested the FERPlus dataset on the popular facial recognition model DeepFace and analyzed DeepFace’s performance in identifying seven dis- tinct emotions across various races and genders. Through the evaluation of True Positive Rates (TPR), False Positive Rates (FPR), True Negative Rates (TNR), and True Postive Rates (TPR) across demographic segments, our findings revealed a consistent perpetuation of biases by both the technology and the dataset. Our findings underscored the urgent need for a more systematic approach to mitigating biases.
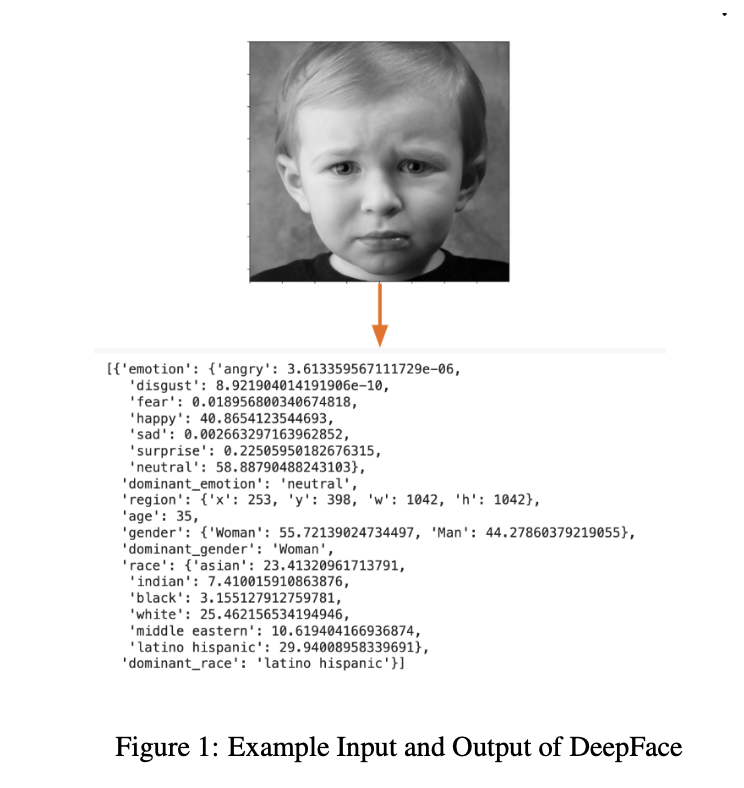
Facial expression recognition, a key application of artificial intelligence, plays a vital role in various societal domains such as online interviews (Zetlin, 2018), personalized avatars (Borak, 2023), and the surveillance of prisons and detention centers where violent behaviors are common (Standaert, 2021). Despite its widespread adoption, facial expression recognition models, including the popular open-source DeepFace (Serengil & Ozpinar, 2021) can contain biases associated with gender, race, and age. In this project, we focus on investigating potential biases within DeepFace by assessing its performance on facial expression recognition using the FERPlus dataset (Barsoum et al., 2016). We aim to evaluate the biases of DeepFace using metrics such as False Negative Rate (FNR) and False Positive Rate (FPR), specifically addressing faces of different genders and races.
Although the decisions made by facial expression recognition models seem small compared to credit evaluation models or probation decision algorithms, understanding the harm of bias in facial expres- sion recognition models is increasingly critical today. Errors made by these algorithms have the potential to reinforce existing biases against specific racial, gender, and age groups. Biased recogni- tion can lead to financial losses, hinder the initiation of crucial mental health interventions, and even pose risks of injuries from unexpected assaults.
By comparing potential disparities in the accuracy of expression recognition of DeepFace between different genders and races, we aim to identify any systematic biases in DeepFace and analyze the underlying causes of these biases. This research will contribute to the ongoing efforts to enhance the fairness and reliability of facial expression recognition technologies, ensuring their responsible and equitable deployment in society for all.
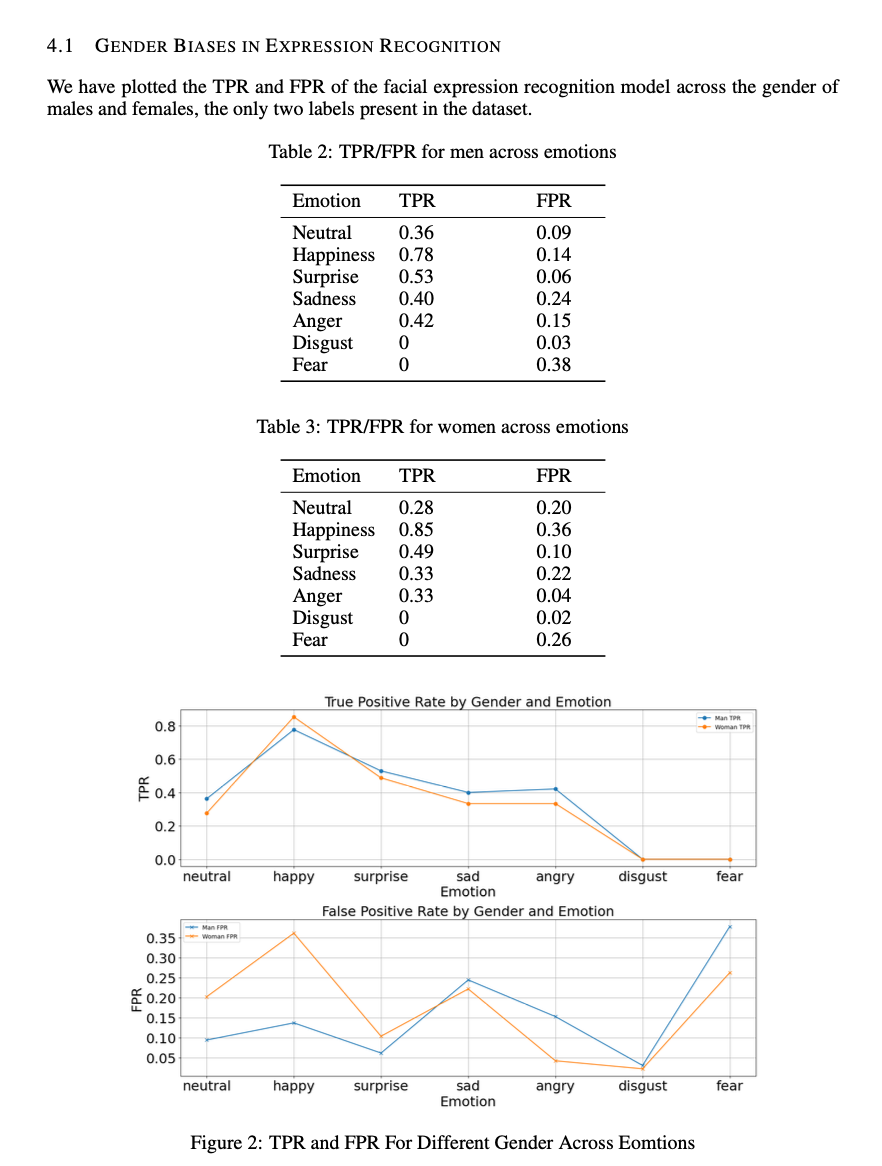
From the plot, several key observations can be made. First, the TPR of emotion recognition is generally higher for men than women, demonstrating that it does not perform equally well across gender groups. We observe the emotion ’happy’ in which the woman’s TPR is higher than that of the man’s TPR. This difference suggests that the model may have been trained on a dataset where expressions of happiness in women are either more frequent or more distinctly represented than in men. This could result from a bias in the dataset towards certain types of facial expressions or emotional displays in women. The higher accuracy in recognizing happiness in women could reflect societal stereotypes where women are often expected to show more openly positive emotions like happiness. If the training data for the model disproportionately represents women smiling or showing happiness, it could lead to this bias. On the other hand, the FPR graph exposes stereotypical representations of men and women across the misinterpretation of certain emotions. High FPR for emotions like anger and fear in men, or happiness and surprise in women, might reinforce stereotypes or lead to misinterpretation in critical situations. The higher FPR for happiness in women and anger in men may inadvertently reinforce gender stereotypes where women are often stereotypically perceived as more emotional or prone to happiness, while men are frequently characterized as more likely to express anger. If an emotion recognition system incorrectly identifies these different emotions more frequently in each gender, it can perpetuate these stereotypes when put into use.
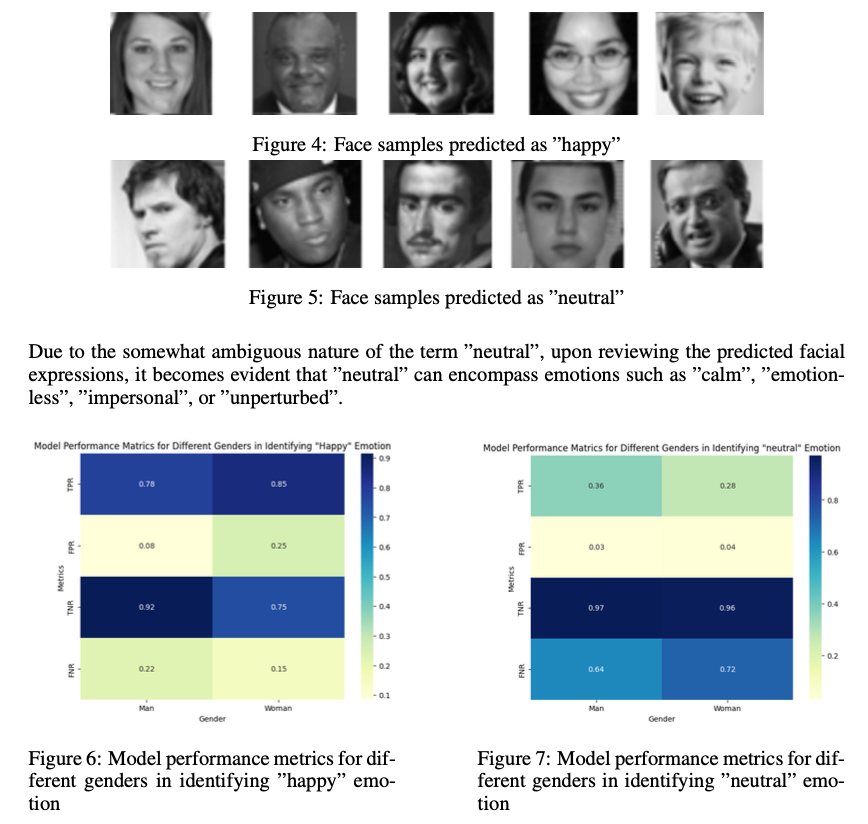
Given the highest accuracy of the ”happy” emotion and the predominant presence of ”neutral” within the predicted faces, we have chosen to focus our analysis on these two primary emotions (Fig.4, Fig.5). Delving into these emotions as case studies, we aimed to investigate potential gender biases. To comprehend the model’s performance in identifying these emotions, we generated heat maps illustrating the true positive rate (TPR), false positive rate (FPR), true negative rate (TNR), and false negative rate (FNR).
In the identification of the ”happy” emotion, the Fig.6 shows that the TPR exhibits a higher value for females (0.85) compared to males (0.78). This suggests that when it comes to correctly identifying the ”happy” emotion, females are more likely to be accurately recognized as experiencing happiness compared to males. Conversely, the TNR is notably lower for females (0.75) in contrast to males (0.92). The higher TNR for males suggests that the model more accurately identifies the absence of happiness in males. However, this could result in more instances of misclassifying unhappy female faces as happy. On the other hand, in identifying the ”neutral” emotion(the Fig.7), there is a lower TPR for females (0.28) compared to males (0.36). Additionally, the FNR is higher for females (0.72) compared to males (0.64). The lower TPR for females implies that females are less likely to be correctly identified as experiencing a neutral emotion. The higher FNR for females suggests they are more likely to be incorrectly categorized as not experiencing a neutral emotion when they actually are.
The model appears more proficient at identifying ”happy” emotions overall, yet disparities emerge when comparing the gender-based results. There are several reasons that can explain these biases. First, the FERPlus dataset contains an imbalance in the representation of ”neutral” and “happy” ex- pressions across genders. In the original dataset, for the identified faces, there are 830 faces of men labeled as neutral, whereas only 324 women were labeled as neutral. Second, there could be differ- ences in how males and females typically express certain emotions through facial cues. If the model is trained on expressions that are more commonly associated with one gender, it might perform bet- ter for that gender in recognizing those emotions. Additionally, the algorithms used for emotion recognition might inherently favor certain facial features or expressions that align more closely with one gender’s typical expressions, leading to differential accuracy in emotion identification.
The consequences of facial emotion recognition bias indicated above, especially when more women are misidentified as displaying positive emotions and more men are misidentified as calm or imper- sonal, can lead to social problems. Biases that consistently misidentify women as displaying posi- tive emotions, even when they’re neutral or experiencing negative emotions, can reinforce societal stereotypes that expect women to be more cheerful or emotionally positive. Similarly, misidentify- ing men as calm or impersonal irrespective of their emotions can reinforce stereotypes that expect men to be stoic and unemotional. Misidentifications can lead to biased outcomes if these biased emotion recognition systems are used in crucial decision-making contexts like job interviews, promotions, or legal proceedings. For ex- ample, if a woman’s neutral expression is consistently interpreted as emotional, it might influence hiring decisions, leading to biased selections.